What Is Credit Decisioning In Lending?
Credit companies deal with risk daily, and credit decisioning is the gatekeeper that is supposed to keep the bad risk out of the business equation. Traditionally, credit companies have deployed complex statistical models that account for multiple variables constituting a credit applicant's profile to assign an internal score, which links the degree of risk that a loan represents in the real world to the company's business rules. Done manually, credit decisioning comes at long and expensive person-hours and falls short of the business needs of operating in today's economic environment by accumulating risk opaquely and eroding control in the enterprise. The Bank for International Settlements now advocates for information and analytical systems that can help bankers and lenders to monitor and assess credit risk in addition to a reasonably granular composition of the credit portfolio.
Challenges With The Traditional Ways Of Credit Decisioning
Traditional lending journeys take the user across approximately 50 different screens and take the user to the approval or disapproval state in several days. Despite being front-office digital, such lending journeys are fraught with manual processes that leverage legacy software and fragmented approaches to data aggregation at the point of credit decisioning. Being human-intensive, such models cost companies a fortune in the process of scaling up and often fail to capture applicants without a verifiable credit history. Beyond this, long waiting periods also result in higher dropout rates, low satisfaction, and reduced business value for the stakeholders.
How Is It Hurting The Fortunes Of Lenders?
Traditional means of loan decisioning can stunt the growth of a lending company in more than one ways:
- A misfit in the digital world- Manually-driven credit decisioning processes do not fit in today's digital context. Customers expect money instantly and physical goods on the same day or the next.
- Uncaptured, untapped value- Relying on systematic financial and credit histories, traditional loan decisioning frameworks exclude several potential points of sale and user personas from the company's radar.
- A digital illusion- Lurking behind seamless frontends, traditional lending not only comes at a higher cost but also bottlenecks the rate of growth and the possibility of upscaling.
- Lack of visibility- In addition to being error-prone and subject to bias, manual decisioning also brings opacity to the enterprise's overall risk exposure.
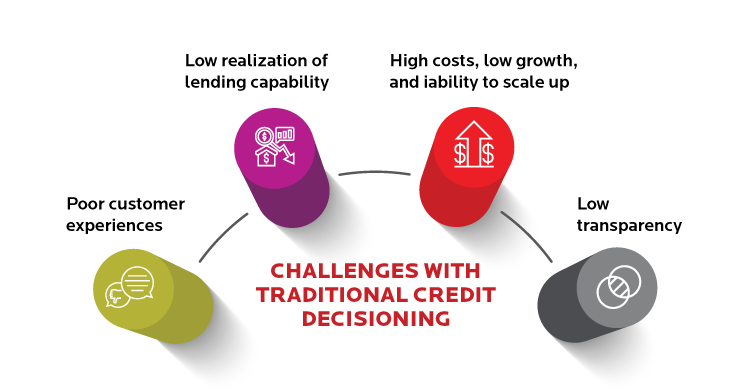
Top challenges with traditional credit decisioning
Lending Digital Transformation: Powered By AI And Automation
How it works
Artificial Intelligence and automation have explored the frontiers of profitability, efficiency, costs, and end-user experience in the lending landscape. With customers' footprints now distributed around the enterprise's digital presence and various social networks, credit decisioning is taking a new turn. Leading lenders leverage unstructured and structured data from these footprints in conjunction with third-party data sources to train rule inferring models with machine learning credit scoring techniques like support vector machines, random forests and ensemble learning, and hybrid genetic algorithms for automatically tuning the model and refining feature selection strategies. Trained for over 99% accuracy, such models can eliminate bias and error and increase churn volume while reducing the need for human touch across most cases.
In conjunction with AI, automation can bring speed to the application-to-disbursal process and help tame the low-complexity application cases at minimal resources. However, some of the best automation implementations automate 70-80% of the cases - moreover, automation becomes challenging in high-complexity commercial lending portfolios. Relying on front-facing portals for automating the collection, warehousing, processing, and decisioning, end-to-end automation can help create new value propositions like instant processing and pre-approved loan offerings.