And the stakes are extremely high for lending banks -- inaccurate assessments can cost organizations sizeable amounts. This is further intensified by sub-optimal underwriting, inaccurate portfolio monitoring methodologies, and inefficient collection models.
Clearly, it is imperative for banks to adopt smarter models of credit assessment that can parse huge volumes of data in truncated timelines, dynamically altering risk profiles as per real-time data. This is where Artificial Intelligence (AI) and Machine Learning (ML) could play a major role.
These technologies are able to learn from complex datasets and become incrementally more accurate over time. Further, the need for human data science expertise and analysts’ efforts is also minimized, as AI & ML models can “black box” the underlying technology to show only the final insights.
The State of AI & ML Application in Credit Risk Assessment
AI & ML technology could find a plethora of use cases in the BFSI sector, and risk management is at the top of this list. Between 2017-18, the number of organizations using AI more than doubled, and 40% of financial services firms are applying it to risk. This is because AI & ML could add genuine value across the credit value chain, starting from the initial underwriting process to risk measurement and analysis, until deciding on the final maximum exposure. Some of the key use cases that would be addressed are:
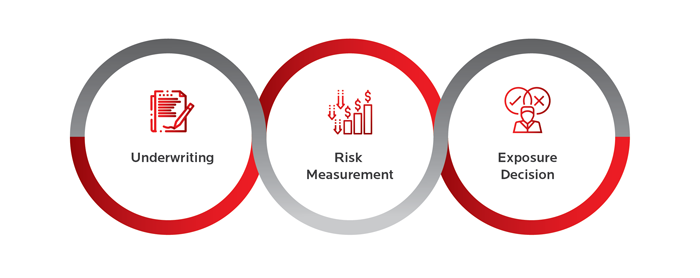
Assessing risk for individual customers
For example, the majority of applicants in any borrower group will comprise non-defaulting individuals; only a very small set will be defaulters. As a result, a sample of bad customers going into the credit dataset could cause imbalance and skew results if banks are using traditional analytical models. Because of performance degradation caused by this gap, the predictive insights are inaccurate, and the bank loses out on viable business opportunities.
An ML model, like the Artificial Neural Network, would create discrete clusters of datasets and apply merging methodologies to figure out if a specific customer should be offered a loan. Instead of merely looking at the mean values, ML creates majority and minority clusters and merges them to create a diverse dataset, reflecting the real on-ground picture.
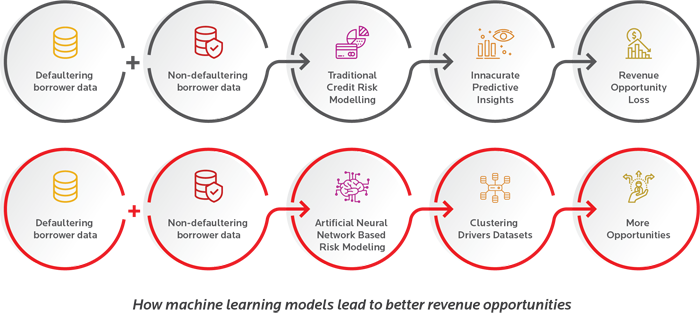