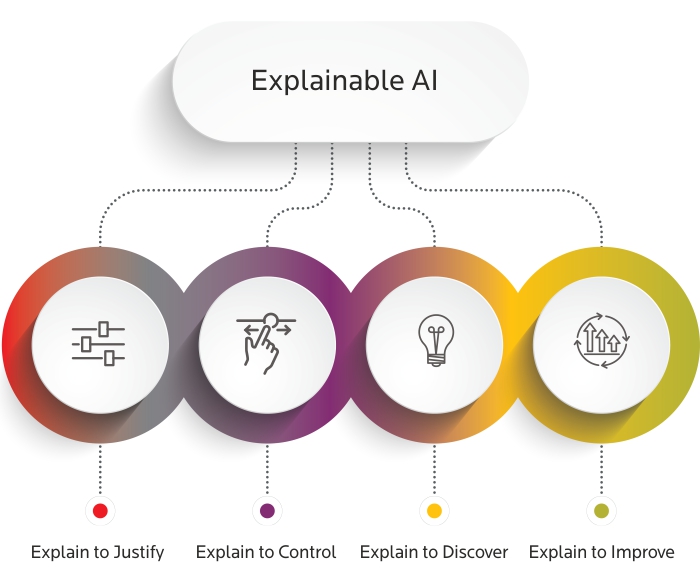
What is Explainable AI?
Explainable AI (XAI) can be considered the collection of processes that help the developer and the users understand and interpret the results.
Developers utilize the XAI results to understand the potential bias in the model and improve the model performance. Users can trust and believe the AI model's inference results as it comes along with the interpretation of the inference. 'Code confidence' usually refers to how much the developer/user has confidence in the AI model's inference. Below questions are hard to answer with currently utilized 'Black Box' like models.
- How do users trust the AI model in an autonomous vehicle to make the right decisions in a high traffic scenario?
- How to make the doctor trust the inference of the AI model diagnosing a particular disease?
Utilizing an XAI solution to explain the results could help the developer provide the necessary code confidence for the end-user.
Model Explainability is a prerequisite for building trust and adoption of AI systems in high-stakes domains requiring reliability and safety, such as healthcare and automated transportation, and critical applications including predictive maintenance, exploration of natural resources, and climate change modeling.
AI vs. Explainable AI
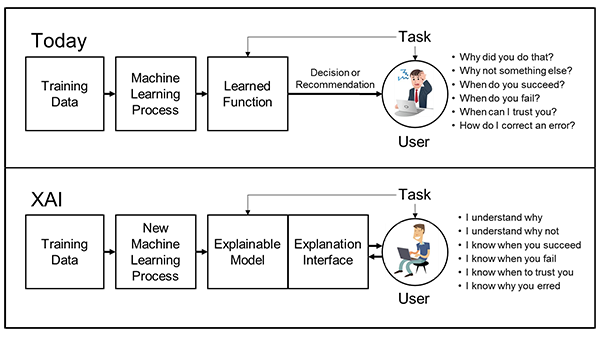
Source: DARPA
The image provides a visual representation of XAI and how it potentially affects the end-user.
XAI solutions have various advantages, which brings them to the spotlight and highlights the need for quicker large-scale adaption worldwide. Regulations (Example: Article 22 of GDPR) give the rights to the individuals to demand an explanation on how an AI system has made a decision that can impact them. The algorithmic Accountability Act of 2019 mandated the companies to perform and provide a risk assessment report for the risks posed by automated decision-making systems based on accuracy, bias, fairness, privacy, security, and discrimination.
Benefits of Explainable AI (XAI)
- Reducing Cost of Mistakes: Decision-sensitive fields such as Medicine, Finance, Legal, etc., are highly affected in the event of wrong predictions. Oversight over the results reduces the impact of erroneous results & identifying the root cause leading to improving the underlying model.
- Reducing Impact of Model biasing: AI models have shown significant evidence of bias. Examples include gender Bias for Apple Cards, Racial Bias by Autonomous Vehicles, Gender, and Racial bias by Amazon Rekognition. An explainable system can reduce the impact of such biased predictions cause by explaining decision-making criteria.
- Responsibility and Accountability: AI models always have some extent of error with their predictions, and enabling a person who can be responsible and accountable for those errors can make the overall system more efficient
- Code Confidence: Every inference, along with its explanation, tends to increase the system's confidence. Some user-critical systems, such as Autonomous vehicles, Medical Diagnosis, the Finance sector, etc., demands high code confidence from the user for more optimal utilization.
- Code Compliance: Increasing pressure from the regulatory bodies means that companies have to adapt and implement XAI to comply with the authorities quickly.
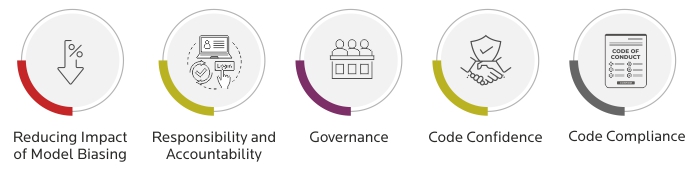
Benefits of Explainable AI