Simultaneously, the number of man-hours that maintenance operations required led cement manufacturers into a double-edged loss - where production capacity was being lost, quality compromised, and cost of reliability climbing ever higher. Predictive maintenance is essentially a collection of processes, frameworks, and technologies that manufacturers now use to cut through this perpetual cost loop.
How does predictive maintenance work in cement manufacturing
Leveraging interconnected devices, lightweight sensors, public clouds, and cutting-edge digital technologies like artificial intelligence (AI) and IIoT (Industrial Internet of Things), predictive maintenance enables cement producers to move away from a reactive and/or a preventive approach to keeping the critical machinery up and running in the production plant at minimal cost and with little to zero human touch. At the most basic level, predictive maintenance relies on sensors placed on, around, or within a machine - say, on the edge of a rotor - that log a critical value reflective of the machine's performance.
Throughout implementation, critical failures related to a machine are tagged, and the data collected from the sensors is then analyzed by advanced machine learning algorithms. While some solutions come with intelligence hardcoded into the system, others evolve by learning from their context. However, spotting the faults before, or as they occur, is only a part of the problem - predictive maintenance is usually supported by a team that leverages this information to take actions or decisions that translate into business value.
How to implement predictive maintenance in cement manufacturing
AI in predictive maintenance
AI essentially serves as the brain of a predictive maintenance platform. Since predictive maintenance relies on data collected from sensors that record the critical parameters about a machine in real-time, the problem of predicting uptime is modeled in different ways under various approaches. For instance, some systems view fault prediction as a classification (or, more precisely, an anomaly detection) problem, while others attempt to minimize the mean error in predicted vs. actual uptimes. In general, AI allows predictive maintenance systems to mimic human-like capabilities, infer a fault, and notify your maintenance engineers about things that might go wrong.
Predictive maintenance and machine learning
Machine learning is a subset of AI techniques that enable the discovery of outliers within a stream of data or instruct algorithms to work a certain way based on a result outcome. For instance, reinforcement learning techniques' reward' an algorithm if it correctly predicted the vibration signals from a kiln roller due to misalignment or excess lubrication on the kiln shell. Machine learning algorithms analyze several different features such as maintenance history, error logs, static device information, and real-time environment variables to detect deviant behavior and attempt to diagnose the root cause based conditions of breakdown.
Predictive maintenance and iot
At the fundamental level, predictive maintenance systems are made possible through various sensors that log data related to an environment variable such as temperature, humidity, vibrations per second, or only images and videos of a target frame in real-time. Streams of data from these sensors are sent to data lakes - where they are processed, logged, and analyzed. Also called the Internet of Things, this interconnected mesh of devices are the eyes and ears of predictive maintenance systems. But where can cement manufacturers benefit from predictive maintenance systems?
A North American cement factory leveraged 5000 sensors and 50 computers to maintain the production machinery with just one operator. Here are some examples with which such levels of efficiency can be built into low-touch systems.
Predictive maintenance use cases in cement manufacturing
Vibration analysis
The cement factory consists of many aggressively moving pieces. The machinery is subjected to particles of varying size, often 6-40 microns in diameter, which can cause bearings, shafts, and seals to wear faster, resulting in failure of critical equipment. Vibration analysis is made possible through sensors that convert force fluctuation from vibrations into electrical signals of proportional strength - which are then analyzed by machine learning algorithms that are continually monitoring for anomalies in the vibration signals. In the cement industry, vibration analysis can monitor motor and gearbox assemblies in kiln drives, crushers, screens, conveyor belts, raw mills, separators, and blowers.
Anomaly detection
In the cement industry, the prediction of potential faults is premised because the real-time data streams originating from the machine's operating conditions carry data about the manner(s) in which a machine ages or loses function and how the environment variables move before and during those failures. Anomaly detection algorithms look for these signals in the real-time data streams - Mahalanobis Distance (MD) and one-class support vector machine (OCSVM) algorithms have shown the most promise in successfully diagnosing manufacturing systems. Anomaly detection is an approach to machine learning problems - and as a result, it can be used on any type of data - even for estimating clinker quality by capturing the spectral profile of the inside of a kiln through an image.
Kiln activity monitoring
Many cement producers over-deliver on their quality SLAs due to their inability to precisely track the quality of a batch due to the difficulty in monitoring the clinker formation due to environmental factors. Some cement manufacturers have deployed radiation thermometers to accurately track the environmental variables like temperature on the inside of the kiln. Others have deployed neural network soft sensors to accurately predict the clinker quality of a batch and thermal imaging sensors to map the best and worst-case scenario temperature profiles across the kiln's critical regions.
Automated capture of maintenance done via newly fitted sensors for Air Filters & Implosion / Explosion Flap
Cement producers are already seeing conversations on Cement 4.0 being a green manufacturing paradigm - but many still rely on guesswork for dust collector maintenance at their plants and are unable to fix filter leaks until visible emission plumes violate their air permit. Some manufacturers and cement tech partners have suggested using triboelectric bag leak detectors to record a leak within seconds of its formation and help producers keep their plants within the emission violation norms without attracting unwanted regulatory attention.
Silo maintenance tracker and maintenance scheduling of critical equipment like pressure relief valves
Most cement manufacturers still leave their workforce with a safety checklist that directs them to conduct roof beam inspections, burgeoning walls, or examining a silo's degeneration. However, this degeneration does not happen overnight. In fact, several silo degradation and failure-related cases could have been predicted by observing these basic safety signs. Moreover, temperature sensors, pressure transducers, displacement sensors, and flow transmitters can be used to monitor control valve performance. Some researchers have already developed a state-of-the-art anomaly detection system for pneumatic actuators in control valves using Artificial Neural Networks to predict the type of cylinder leakage and forecast the position of a breach.
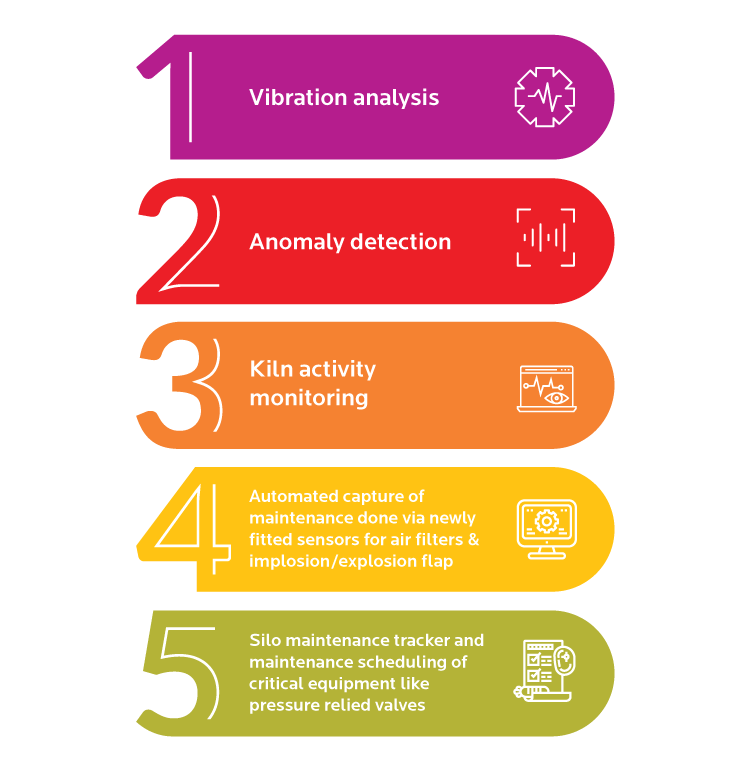
Five use cases of predictive maintenance in cement manufacturing
Why predictive maintenance is essential in cement manufacturing
While the 20% maintenance cost reduction of predictive maintenance is not difficult to spot, cement manufacturers must also consider the lateral benefits that come with such systems:
Predictive maintenance systems create a superior employee experience by connecting instrumentation experts with on-site engineers and reduce the need for human touch.
Producers can achieve better reliability with half-sized teams - which means more uptime, reduced uncertainty of failure, and cost savings.
Hyper-optimized energy consumption, better adherence to regulatory norms, and accelerated production.
Predictive maintenance is slowly becoming the currency of competitive advantage in this industry - it is no surprise that BCG has called it the first 4.0 priority in this industry.